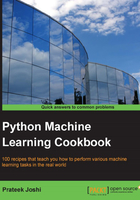
上QQ阅读APP看书,第一时间看更新
Splitting the dataset for training and testing
Let's see how to split our data properly into training and testing datasets.
How to do it…
- Add the following code snippet into the same Python file as the previous recipe:
from sklearn import cross_validation X_train, X_test, y_train, y_test = cross_validation.train_test_split(X, y, test_size=0.25, random_state=5) classifier_gaussiannb_new = GaussianNB() classifier_gaussiannb_new.fit(X_train, y_train)
Here, we allocated 25% of the data for testing, as specified by the
test_size
parameter. The remaining 75% of the data will be used for training. - Let's evaluate the classifier on test data:
y_test_pred = classifier_gaussiannb_new.predict(X_test)
- Let's compute the accuracy of the classifier:
accuracy = 100.0 * (y_test == y_test_pred).sum() / X_test.shape[0] print "Accuracy of the classifier =", round(accuracy, 2), "%"
- Let's plot the datapoints and the boundaries on test data:
plot_classifier(classifier_gaussiannb_new, X_test, y_test)
- You should see the following figure: