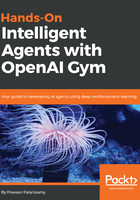
上QQ阅读APP看书,第一时间看更新
Markov Decision Process
A Markov Decision Process (MDP) provides a formal framework for reinforcement learning. It is used to describe a fully observable environment where the outcomes are partly random and partly dependent on the actions taken by the agent or the decision maker. The following diagram is the progression of a Markov Process into a Markov Decision Process through the Markov Reward Process:

These stages can be described as follows:
- A Markov Process (or a markov chain) is a sequence of random states s1, s2,... that obeys the Markov property. In simple terms, it is a random process without any memory about its history.
- A Markov Reward Process (MRP) is a Markov Process (also called a Markov chain) with values.
- A Markov Decision Process is a Markov Reward Process with decisions.